The title is a reference to my favourite of the many quirky poems and toasts originating from my adopted home country of Scotland. It is an ode to the spirit and exceptionalism common of the inhabitants of this rain-lashed northern tip of a small island that has allowed their culture to survive and thrive through the ages. Exceptionalism in cultures is one thing but I found it somewhat surprising recently to be told by a Senior Geologist working for a major Operator that, in the world of upstream oil reservoirs, exceptionalism held true as well. His view was that there was no such thing as an “analogue” in the world of an oil field and that, as every field and reservoir has a totally unique combination of the hundreds of important characteristics that influence development potential, only a ground-up study of each field and reservoir could lead to insights that were accurate enough to be of use. Whilst I am pleased to say that this is the most extreme level of myopic thinking I have encountered, and most technical professionals would concede that there is at least some value in studying fields similar to their own, in my experience there is still a fair degree of the “Wha’s like us?” attitude prevalent in us when thinking about our reservoirs.
In regards to uniqueness there is a somewhat surprisingly good analogy to be drawn between oil fields and us, or specifically the human genome that defines us. Each of us has about three billion base-pairs that form the building blocks of our DNA, each with a totally unique combination of base-pairs inherited 50% from our Father and 50% from our Mother. Even identical twins develop post-partum random mutations in their DNA that set them apart from each other. In this sense we are much like oil fields, defined by a unique combination of many factors which all have a complex interplay with each other and their surrounding environment. As such my friend the Senior Geologist might be tempted to claim that the behaviours of each of us could only be understood and predicted by exhaustive analysis of our individual genome and a full review of our personal history. The very existence of the scientific field of Behavioural Science (and the success of the Facebook, Google and Amazon algorithms) puts some pay to this view.
So if we can assert that there is such a thing as an analogue to our field it follows that there may be something to be learned from understanding it. Happily, studying analogues for clues to our field’s character and likely potential is orders of magnitude cheaper than drilling wells or shooting seismic. Why then (with the notable exception of front-end exploration where no field-specific data is present) is an analogue study not the first thing we typically do when analysing a field? Again, the answer may in part lie in DNA – this time our collective DNA as a professional group.
The route to becoming a subsurface professional puts a high degree of selection pressure on one’s ability to be scientifically accurate and pay attention to fine-grained technical details. This skill is a prerequisite of being able to construct effective geological or simulation models, not to mention being of value in all the other aspects of project planning, economics, HSE, risk analysis (etc.) that are needed for a successful project. In short, engineering & geoscience attracts details people in the first place and rewards that behaviour throughout a career.
Unfortunately, no analogous selection pressure is present on our ability to take a big-picture view and ask ourselves “does that model I just built make real-world sense?”. How often after University Assignments or Industry Gate Reviews have you been asked to justify the forecasts of your model by ensuring they fell within the performance range of the 5, 10 or 100 closest analogues?
Given the tens of thousands of fields have been developed in the past and the huge monetary and time investments involved in putting together detailed models, it is perhaps surprising that this question is not the first question asked at any review. After all, who really cares if the Corey exponent is 3 or 4 if the overall results of the model don’t align with what we have seen 100 times before in similar circumstances (or we don’t have a convincing reason to explain any such variance). Whilst it is surprising, there are I think three key factors underlying why this question is not asked more often:
- The afore-mentioned cognitive bias of professionals to start (and end) with the details;
- Increasing project time pressures being applied to technical departments with diminished staffing levels mean the detailed model build takes up all available time and;
- The fact that most technical workflows were designed (and most managers cut their teeth) in a world where getting analogue information was hard and time-consuming where-as now it is easy and instantaeneous
The worst examples of analogue studies I see are those carried out after the completion of detailed models with corporate deadlines, such as gate reviews, looming. The tendency in these scenarios is to, even subconsciously, cherry-pick the analogue data that supports our existing model results. The alternative is just too painful in most circumstances – telling the boss that the model we have doesn’t really match the prevailing global trends and we need to push back deadlines whilst we cycle back on months of expensive modelling work. A route to promotion it is not… so better to trust in the model and hope for the best. Would it not have been better to have an idea of what results we should expect before launching into the details, so we can be more confident?
To make matters worse, all-to-often I see analogue studies conducted informally (by the afore-mentioned time-stressed professionals) with bespoke internet research limited to only a handful of fields with analysis limited to basic parameter comparison tables.
Tools such as bMark™ mean that we can do so much better as an industry and start to set an expectation where considering the big picture is just as important as getting the details right. Empowering staff with global datasets and the ability to analyse them quickly so as to fit into project deadlines allows us to overcome our collective cognitive bias towards detail and allows much more effective optimisation and QC of the models we build. The force multiplier of such a tool when rolled out across an organization ultimately works to augment skill sets, increase efficiency, and save time and cost in the long run.
So, the next time you run a field project be sure to ask the question – “Wha’s like us”? With the right tools and approach, I wager the answer will not be “Gey Few”!
Until next time.
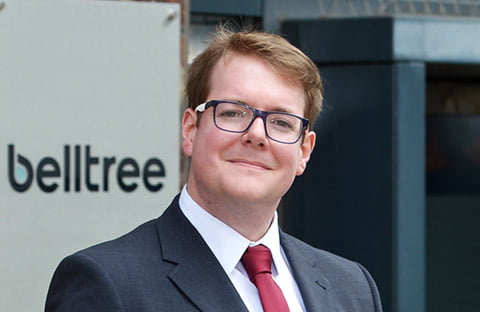